ESR 3: Deploying and Scaling Knowledge Models in Data Science
Panagiotis KourouklidisBritish Telecom (United Kingdom)
Objectives
Data science is playing an increasingly important role in industrial settings and in particular in large enterprises. Managing workforce allocations, improving customer support and improved insight in fault rates are only a few areas in which large enterprises can leverage the significant amounts of data they collect. A major challenge however lies in the development of applications that take advantage of these insights that offer acceptable performance, scalability and longevity.
This challenge emerges from the conventional development practice that is employed in data science research and innovation. The initial stages of a data science project is very much exploratory and research-oriented as at that point the exact potential and use of the available data is still unknown. Using research-support tools and development environments a knowledge model is created that is typically suitable as a proof-of-concept but does not offer the required performance and scalability. Therefore a second development stage is required, typically referred to as down-streaming, which focusses on reimplementing the knowledge
model in a more suitable environment to ensure an application that is production-ready. As this downstreaming stage is currently mostly manual there is a significant impact in time and to the adoption rate of knowledge models, which is further exacerbated by the fact that knowledge models require frequent updates due to changes in the environment and general trends in the data on which they are based.
This project will investigate high-level abstraction languages for LCE knowledge models that are created in data science research and development, in order to help developers downstream such models into scalable, production-ready applications. Developers should not have to deal with the repeated translation of knowledge models to more highly performant technology platforms, but rather focus on creating the infrastructure to accommodate the use of such models in real-world application.
Expected Results
The first objective of the project is to develop a reference model for the transformation of knowledge models to specific target platforms. A core focus of this model is to prevent regression, i.e. ensure functional behaviour, across transformations while facilitating highly scalable applications to be developed with the transformed model. A second core focus of this model is the ability to ensure consistent API black-boxing, meaning that APIs for interaction with the knowledge models can be agreed as a contract. This should make it possible to automate the replacement and deployment of an updated knowledge model inside a (running) application.
We expect a significant reduction of the time required to downstream knowledge models into production applications, with development times potentially reduced 30-50%. Further benefit will be achieved with the automated redeployment of updated knowledge models. Currently due to the overhead involved this is not done, resulting in applications with deteriorating accuracy as time passes. The ability to easily deploy updated models will significantly improve the relevance and accuracy of the applications over a longer period of time.
Publications
-
A Model-Driven Engineering Approach for Monitoring Machine Learning Models. Panagiotis Kourouklidis, Dimitris Kolovos, Joost Noppen, Nicholas Matragkas, Oct. 2021. MDE Intelligence (Models 2021 Workshop)
-
Towards a low-code solution for monitoring machine learning model performance. Panagiotis Kourouklidis, Dimitris Kolovos, Nicholas Matragkas, Joost Noppen, Oct. 2020. ACM/IEEE 23rd International Conference on Model Driven Engineering Languages and Systems, (Virtual Conference) (MODELS 2020)
Supervisors
-
Dimitris Kolovos
Supervision (UY)
-
Nicholas Matragkas
Supervision (UY)
-
Joost Noppen
Supervision (BT)
Secondments
Secondment 1: Collaboration with ESR10 on testing of application within LCEPs.
-
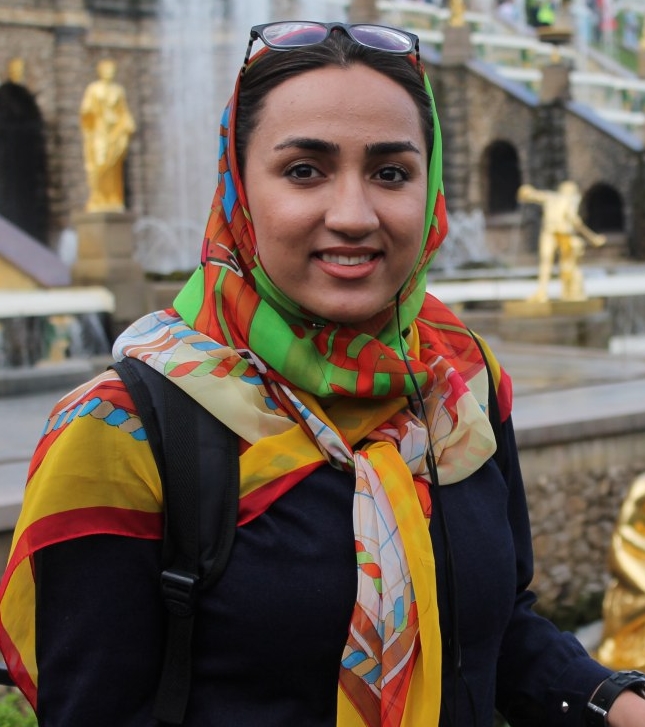
Faezeh Khorram
ESR 10
(IMT)
-
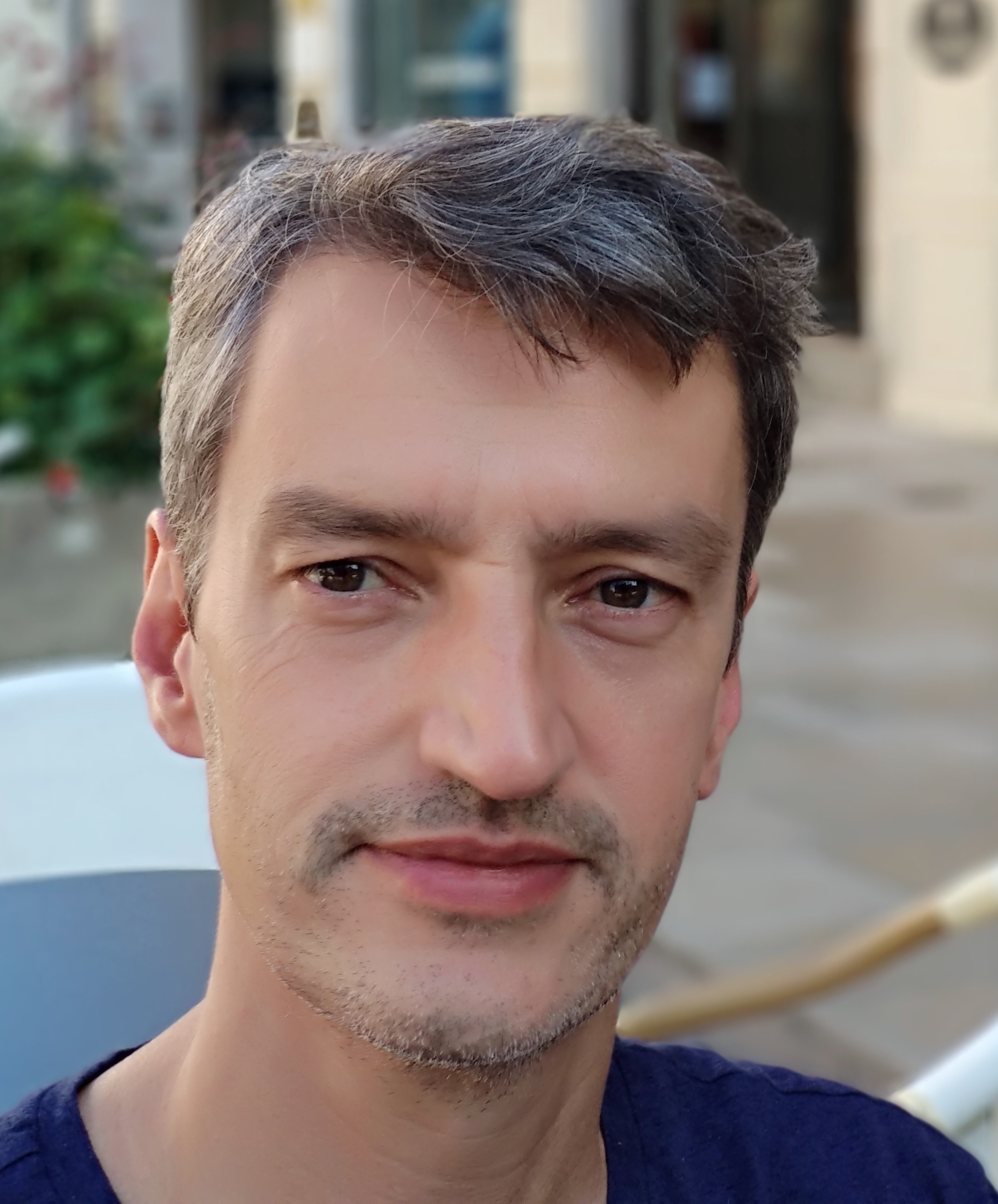
Gerson Sunye
Supervision
(IMT)
-
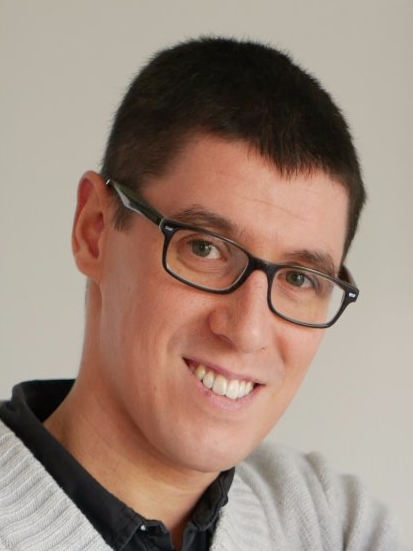
Jean-Marie Mottu
Supervision
(IMT)
Secondment 2: Experimentation, in collaboration with ESR5, on knowledge models from usage traces of low-code mobile applications.
-
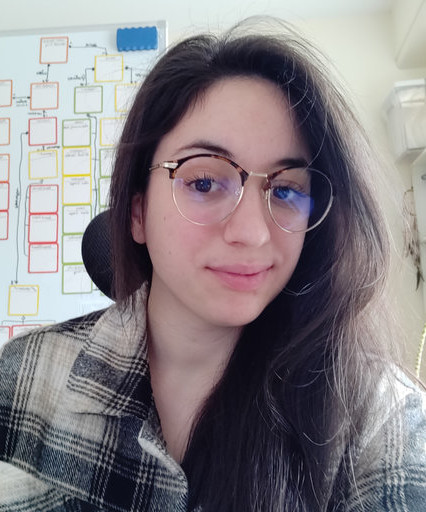
Léa Brunschwig
ESR 5
(UAM)
-
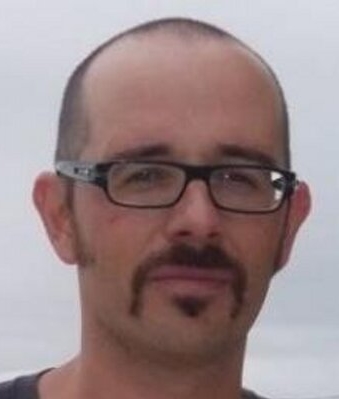
Juan de Lara
Supervision
(UAM)
-
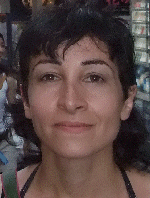
Esther Guerra
Supervision
(UAM)
Will be visited by
-
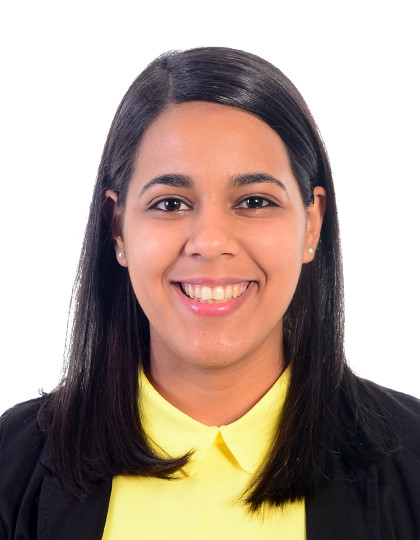
Lissette Almonte Garcia
ESR 1
(UAM)
-
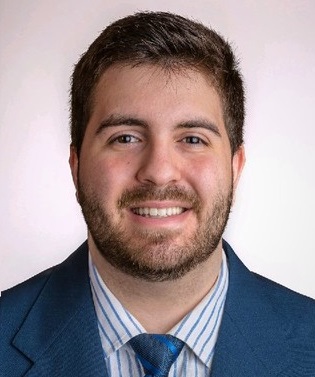
Francisco Martínez Lasaca
ESR 2
(UGD)
Other ESR:
1;
2;
3;
4;
5;
6;
7;
8;
9;
10;
11;
12;
13;
14;
15;
-
Faezeh Khorram
ESR 10 (IMT)
-
Gerson Sunye
Supervision (IMT)
-
Jean-Marie Mottu
Supervision (IMT)
Secondment 2: Experimentation, in collaboration with ESR5, on knowledge models from usage traces of low-code mobile applications.
-
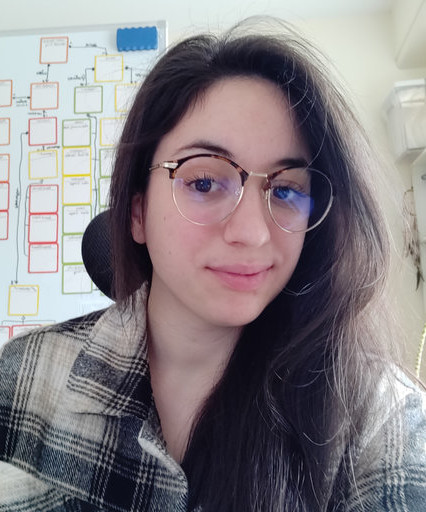
Léa Brunschwig
ESR 5
(UAM)
-
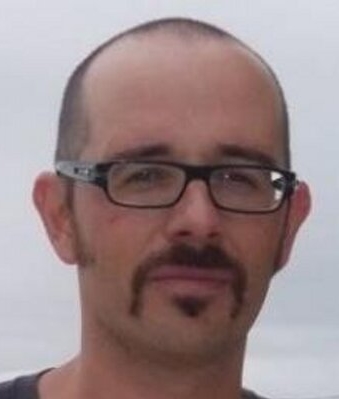
Juan de Lara
Supervision
(UAM)
-
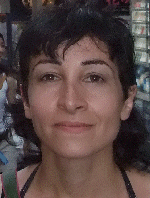
Esther Guerra
Supervision
(UAM)
Will be visited by
-
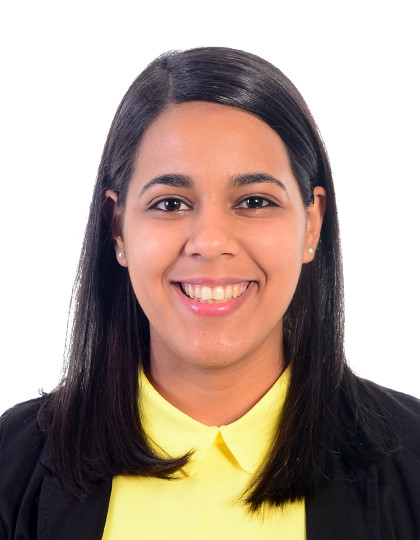
Lissette Almonte Garcia
ESR 1
(UAM)
-
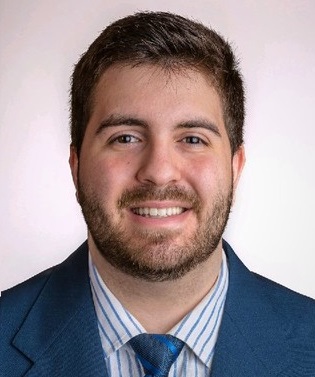
Francisco Martínez Lasaca
ESR 2
(UGD)
Other ESR:
1;
2;
3;
4;
5;
6;
7;
8;
9;
10;
11;
12;
13;
14;
15;
-
Léa Brunschwig
ESR 5 (UAM)
-
Juan de Lara
Supervision (UAM)
-
Esther Guerra
Supervision (UAM)
Will be visited by
-
Lissette Almonte Garcia
ESR 1 (UAM)
-
Francisco Martínez Lasaca
ESR 2 (UGD)
Other ESR: 1; 2; 3; 4; 5; 6; 7; 8; 9; 10; 11; 12; 13; 14; 15;